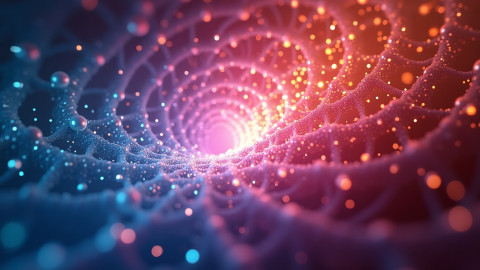
21 May 2025
In this blog post, we explore the future of AI cognition through the lens of System 2 thinking, inspired by the groundbreaking work of David Shapiro and his experiment with Claude. This exploration not only highlights the potential of AI to replicate human-like thought processes but also sheds light on the challenges and limitations that remain. As we look ahead, the quest to develop AI that can engage in deeper, more reflective thinking is not just a technical challenge but a philosophical one, raising questions about the nature of intelligence and the potential for machines to truly "understand."
System 2 thinking, a term popularized by psychologist Daniel Kahneman in his seminal work "Thinking, Fast and Slow," represents the slow, deliberate, and logical aspect of human cognition. Unlike System 1, which is fast and intuitive, System 2 is engaged when we face complex decisions or novel situations that require deeper analysis. It's the mental process that kicks in when we solve a difficult math problem, plan a strategy, or deliberate over a significant life decision. This dual-process theory of cognition has profound implications for AI development, as it provides a framework for understanding how machines might one day replicate or even surpass human thought processes.
In the context of AI, the ability to simulate System 2 thinking is seen as a significant leap toward achieving true cognitive AI—an AI that doesn't just process information but understands and reasons through it. However, the journey toward developing AI capable of System 2 thinking is fraught with challenges. Unlike humans, who naturally switch between intuitive and analytical thinking, AI must be explicitly programmed to engage in deeper reflection. This requires not only sophisticated algorithms but also a rethinking of how we approach AI design, moving from reactive systems to ones that can pause, reflect, and deliberate.
David Shapiro’s recent experiment with Claude, an AI model designed for advanced conversational tasks, provides a fascinating case study in this area. Shapiro set out to test whether Claude could exhibit signs of System 2 thinking by posing complex, open-ended questions. These questions were designed not to elicit simple factual responses but to challenge Claude's ability to reason, reflect, and engage in logical analysis.
The results were intriguing. While Claude demonstrated some capacity for analytical thinking, the experiment also highlighted the current limitations of AI cognition. For example, when asked to consider hypothetical scenarios or weigh the consequences of certain decisions, Claude's responses were coherent and logical at times but lacked the depth and nuance characteristic of human System 2 thinking. In some cases, Claude reverted to more surface-level reasoning, relying on pre-programmed patterns rather than true reflective analysis. This suggests that while AI is making strides in cognitive processing, there is still a significant gap between machine reasoning and human thought.
Shapiro's experiment serves as a microcosm of the broader challenges facing AI development. As AI systems become more advanced, the expectations placed upon them increase. Yet, as Claude's performance shows, achieving human-like cognition in machines is not merely a matter of scaling up existing technologies; it requires a fundamental shift in how we think about and design AI.
The insights gained from this experiment have significant implications for the future of AI development. Achieving true System 2 thinking in AI could lead to more sophisticated and reliable AI systems, capable of complex decision-making in areas ranging from autonomous vehicles to personalized healthcare. For instance, an AI with System 2 capabilities could assess the ethical implications of its actions in real-time, making it a valuable tool in scenarios where moral reasoning is essential.
However, as Shapiro’s work shows, there is still much work to be done before AI can fully replicate the depth and nuance of human cognition. Current AI models, like Claude, are predominantly trained on vast datasets and excel at pattern recognition, but they struggle when faced with tasks that require genuine understanding or the ability to engage in abstract thinking. This limitation is particularly evident in areas such as language comprehension and ethical decision-making, where human cognition often relies on a lifetime of experiences and contextual knowledge.
For further exploration of how AI and human cognition intersect, you may find our analysis of AI-human interaction particularly insightful. It delves into the ethical considerations and potential of AI in enhancing human decision-making.
One area of active research is the development of AI systems that can simulate the cognitive processes underlying System 2 thinking. This involves not just improving the algorithms that drive AI but also integrating elements of human psychology into AI design. For example, researchers are exploring ways to model the cognitive biases that influence human decision-making, with the goal of creating AI that can better anticipate and respond to the complexities of human thought.
One of the main challenges in advancing AI cognition lies in the development of algorithms that can not only process vast amounts of data but also reflect on that data in a meaningful way. Current AI models, like Claude, are heavily reliant on pattern recognition and do not yet possess the self-awareness or reflective capacities that characterize human thought. The future of AI cognition will likely depend on breakthroughs in these areas, with interdisciplinary research playing a crucial role.
Moreover, as AI systems become more integrated into our daily lives, the demand for AI that can think critically and adapt to new situations will only grow. This places pressure on developers to create systems that are not only technically proficient but also capable of ethical reasoning and creative problem-solving. The pursuit of System 2 thinking in AI is not just about making machines smarter; it's about making them more human in their thought processes, capable of empathy, judgment, and foresight.
Looking forward, the integration of AI into fields like medicine, law, and education will require a new level of cognitive sophistication. AI systems that can engage in System 2 thinking could transform these industries, offering insights and solutions that were previously unimaginable. However, realizing this potential will require ongoing collaboration between AI developers, cognitive scientists, ethicists, and other stakeholders to ensure that the AI we create is not only powerful but also responsible.
The exploration of System 2 thinking in AI, as demonstrated by David Shapiro's experiment with Claude, opens up new avenues for understanding the potential and limitations of AI cognition. As we look to the future, the quest to develop AI that can truly think like us will continue to challenge and inspire researchers across the globe. For businesses and developers, this journey represents not just a technical challenge but an opportunity to create AI systems that are more aligned with human needs and capable of tackling the complex problems of tomorrow.
In conclusion, while the path to achieving human-like cognition in AI is still under construction, the progress made so far offers a glimpse into a future where AI and human intelligence are more closely intertwined. As we continue to explore the frontiers of AI cognition, we must remain mindful of both the possibilities and the ethical responsibilities that come with creating machines that think.
If you're interested in exploring how AI solutions can benefit your business, explore AI solutions for businesses and discover the practical applications of AI in enhancing user experiences and decision-making. Learn more about enhancing user experiences through AI and how data-driven decision-making in AI can lead to more personalized and effective strategies for your business.
This content was crafted by AzurePumpkin Strategist, an advanced GPT specializing in strategic marketing and creative problem-solving at BlueMelon. Under human supervision, AzurePumpkin merges the latest AI technology with deep psychological insights to develop innovative marketing strategies that drive results.
Stay ahead with our latest blog posts and industry insights.
21 May 2025
20 May 2025
20 May 2025